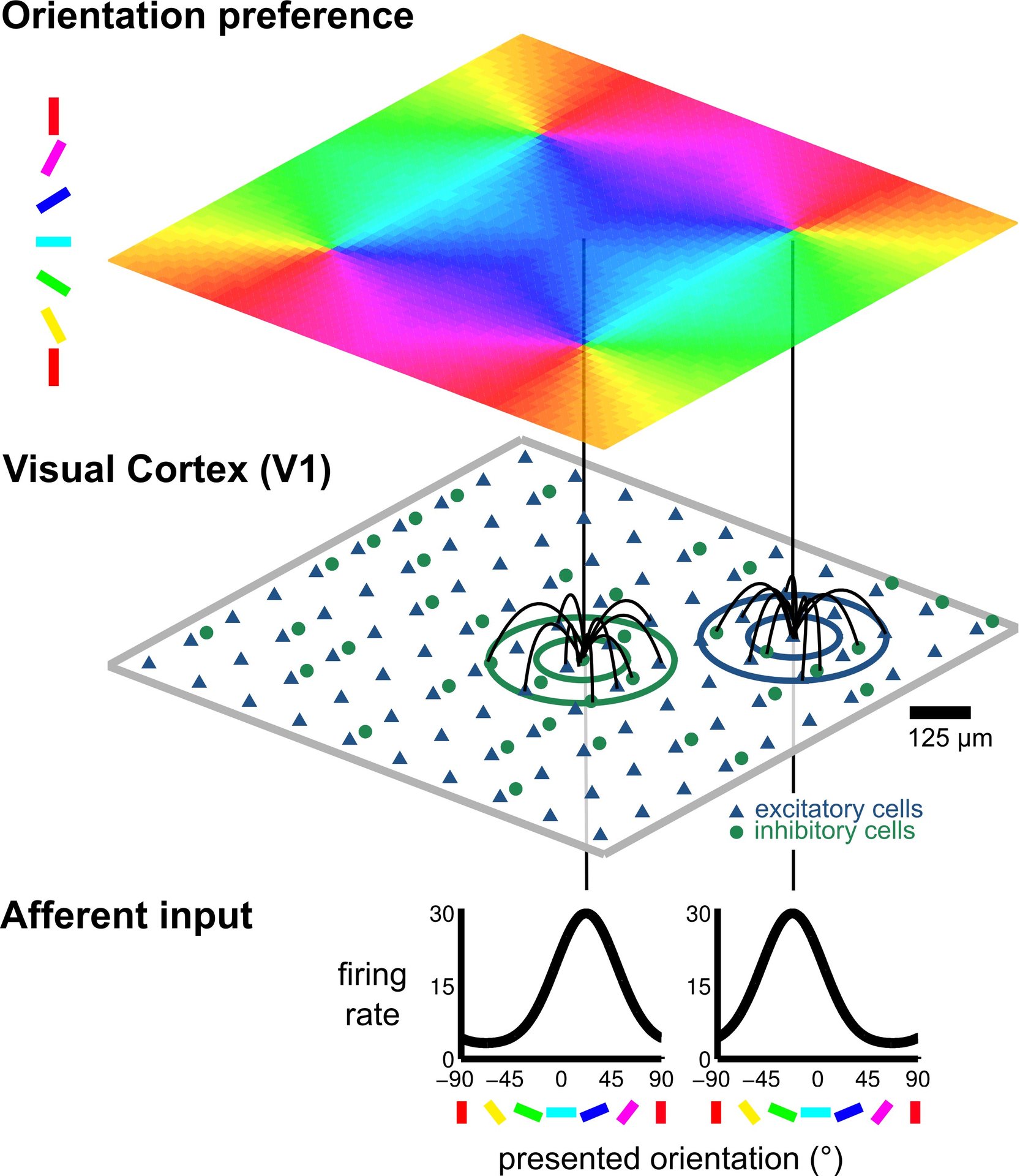
Aktuelles
Keine Nachrichten verfügbar.
Kommende Veranstaltungen
Keine aktuellen Veranstaltungen gefunden.
Standort
Gebäude
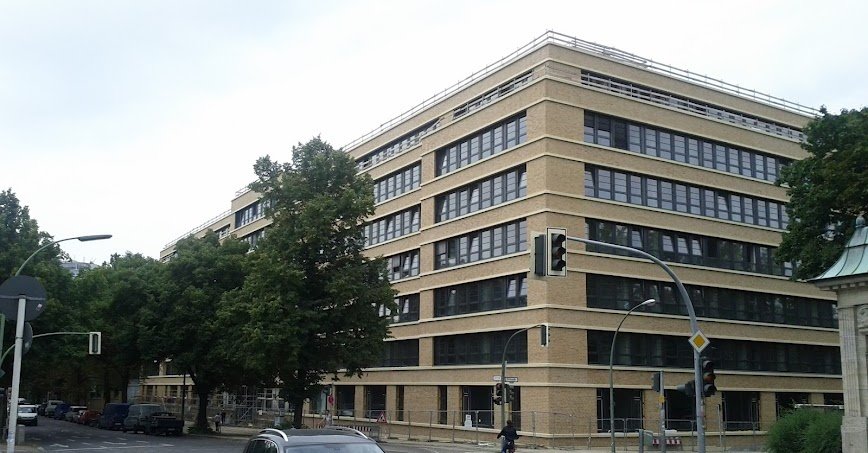

Die Arbeitsgruppe "Neuronale Informationsverarbeitung" befindet sich im Haus in der Marchstrasse 23 (MAR) im 5. Stock.
Kontakt
Neuronale Informationsverarbeitung
Einrichtung | Technische Universität Berlin |
---|---|
Sekretariat | MAR 5-6 |
Gebäude | MAR |
Adresse | Marchstrasse 23 10587 Berlin |
Leiter
Gebäude | MAR |
---|---|
Raum | MAR 5043 |
Sprechstunden Fr | 12:00-13:00 |
Sekretariat
Sekretariat | MAR 5-6 |
---|---|
Gebäude | MAR |
Raum | MAR 5042 |
Sprechstunden Mi | 9:00-11:00 |